-
Courses
Courses
Choosing a course is one of the most important decisions you'll ever make! View our courses and see what our students and lecturers have to say about the courses you are interested in at the links below.
-
University Life
University Life
Each year more than 4,000 choose University of Galway as their University of choice. Find out what life at University of Galway is all about here.
-
About University of Galway
About University of Galway
Since 1845, University of Galway has been sharing the highest quality teaching and research with Ireland and the world. Find out what makes our University so special – from our distinguished history to the latest news and campus developments.
-
Colleges & Schools
Colleges & Schools
University of Galway has earned international recognition as a research-led university with a commitment to top quality teaching across a range of key areas of expertise.
-
Research & Innovation
Research & Innovation
University of Galway’s vibrant research community take on some of the most pressing challenges of our times.
-
Business & Industry
Guiding Breakthrough Research at University of Galway
We explore and facilitate commercial opportunities for the research community at University of Galway, as well as facilitating industry partnership.
-
Alumni & Friends
Alumni & Friends
There are 128,000 University of Galway alumni worldwide. Stay connected to your alumni community! Join our social networks and update your details online.
-
Community Engagement
Community Engagement
At University of Galway, we believe that the best learning takes place when you apply what you learn in a real world context. That's why many of our courses include work placements or community projects.
Health Data Science (MSc)
Course Overview
Health Data Science is a rapidly growing discipline, where skilled people are in extremely high demand from industry due to data-led innovations across the sector. Health related data are generated from many sources including observational studies, clinical trials, computational biology, medical records, individual monitoring devices, health care claims, genetic and genomic epidemiology, environmental health, sports science and climate change.
The aim of the programme is to train a new generation of world-leading health data scientists with the essential statistical and computing skills needed to become data science specialists in the health care, biopharmaceutical and medical technology sectors.
This conversion MSc can be tailored around your skills, experience and interests to provide flexible opportunities for those with either strong quantitative backgrounds, or those with an interest in Healthcare that wish to develop their data science and data analytics skills. Graduates will develop the key statistical and computing skills needed to design studies, analyse, interpret and translate research findings to evaluate health interventions, services, programmes, and policies.
The modules will be delivered by recognised experts in pure and applied health data science including statisticians, bioinformaticians, clinicians, mathematicians, health economists, epidemiologists and computer scientists and invited speakers from Medtech, Pharma and Data Science.
The programme is tailored to allow a wide range of student backgrounds, but primarily to:
- focus skills of those with quantitative backgrounds to needs of the health sector and
- provide biomedical, healthcare and related professionals a path to retrain or upskill in data science.
The courses provide a broad range of skills in statistical modelling, machine learning and clinical research. These skills are brought to fruition in an interdisciplinary capstone research project with either an academic or industry focus.
You will learn about sources of health related data, regulatory environment, ethics, the tools and skills to collate and analyse diverse datasets across various health domains, develop work-ready skills, and understand the varied roles of health data scientists. The wide range of backgrounds and experience of students will create a dynamic learning environment where students can collaborate with peers from different domains to build those work ready skills. On completion you will have the core skills and expertise in the use of statistical data science theory, methods and tools for health and related applications.
Data science skills are in unprecedented demand from many industries, particularly in health care. From precision medicine, to next-generation genomics, to individualised monitoring devices the growth in data collection and data-led decision making is revolutionising health care delivery. If you want to be at the forefront of this revolution in health care in Ireland or globally then this is the MSc for you.
Applications and Selections
Applications are made online via the University of Galway Postgraduate Applications System. Selection is based on a combination of the candidate's academic record, CV including research/professional experience and personal statement (see Supporting Documents website). Applicants may be invited to interview.
Who Teaches this Course
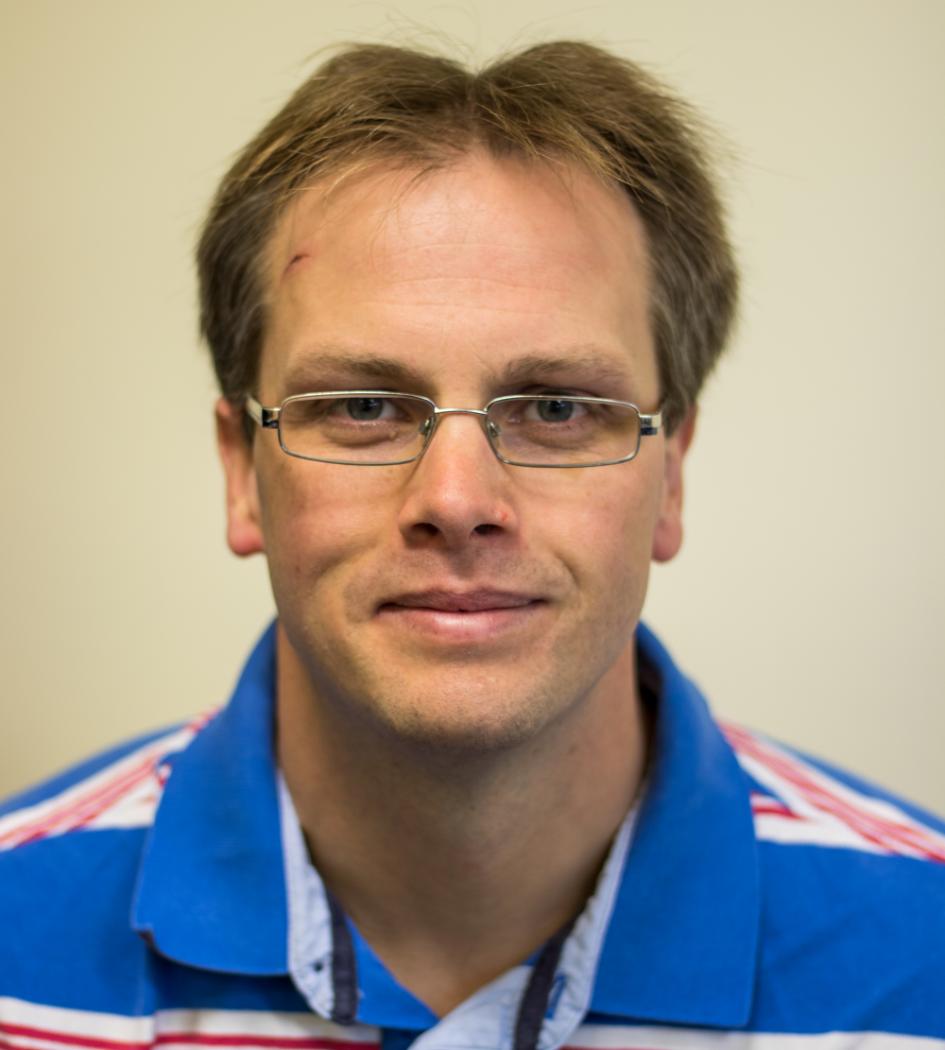
School of Mathematics, Statistics and Applied Mathematics
National University of Ireland in Galway
View Profile
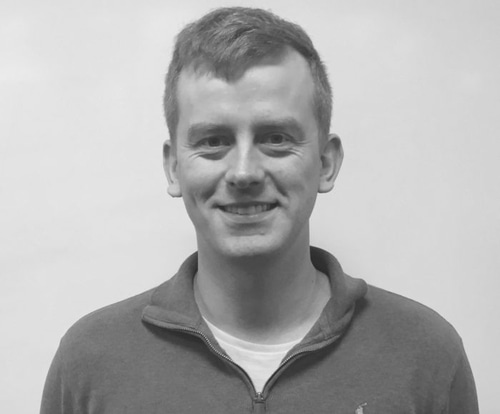
School of Mathematical and Statistical Sciences
University Road
Galway
View Profile
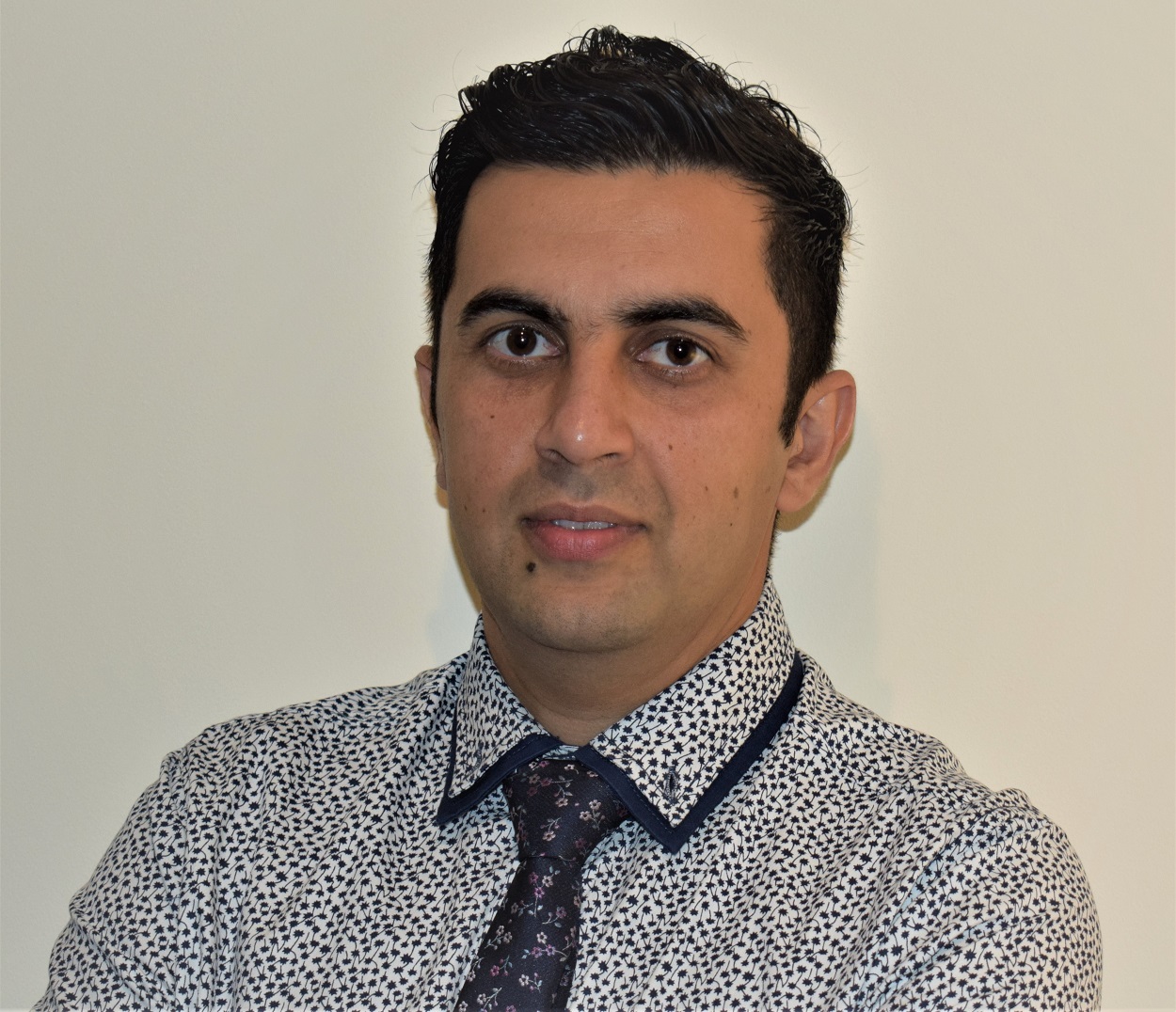
School of Mathematical &
Statistical Sciences
NUI Galway
View Profile
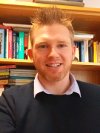
SCHOOL OF MATHEMATICS
& APPLIED MATHEMATICS
University of Galway
View Profile
Requirements and Assessment
Students are formally assessed through a variety of continuous assessment and end-of-semester written examinations. Continuous assessment include written assignments, data analysis projects (including programming), and individual and group presentations. Assessment of the research project includes a literature review and dissertation, as well as an oral presentation.
Key Facts
Entry Requirements
The minimum entry requirement is an upper second class (or equivalent) class honours degree from a recognised University, in a relevant subject (e.g. statistics, health, biological/biomedical sciences, engineering, computer science, psychology) or evidence of achievement at postgraduate level. Performance in the last two years of study will be considered.
Additional Requirements
Recognition of Prior Learning (RPL)
Duration
1 year, full-time
Next start date
September 2025
A Level Grades ()
Average intake
23
QQI/FET FETAC Entry Routes
Closing Date
No set closing date. Offers made on a continuous basis.
NFQ level
Mode of study
ECTS weighting
90
Award
CAO
Course code
MSC-HDS
Course Outline
The learning outcomes for this course include:
- Knowledge and experience of Statistical Data Science tools to analyse diverse health related data;
- Understanding the various sources of health related data and sampling methods for data collection in clinical research;
- The ability to collate, clean and wrangle large diverse health related datasets;
- Being able to identify the appropriate analysis needed and be able to write a statistical analysis plan;
- Developing interdisciplinary work ready skills and the ability to communicate results effectively;
- The opportunity to develop bespoke data science skills as needed for career development.
The 90 ECTS programme will have:
(a) 60 ECTS of taught modules (30 are core and 30 from electives) over both semesters; and
(b) a 30 ECTS research project
The core taught modules are Statistics for Health Data Science, Statistical Computing for Biomedical Data, Clinical Research Design, Statistical Modelling in Health Data Science, Predictive Modelling and Statistical Learning and Causal Inference. The elective modules provide for a broad range of interests across statistical data science, mathematics, bioinformatics, computer science, ethics, health and health economics.
Full-time students will complete the taught modules over Semesters 1 and 2, and complete the research project by the end of the term.
A range of assessment styles will be used across the taught modules, including assignments, individual and group projects, presentations and exams. A key focus will be on the development of work-ready skills for the modern workplace, including assessments using dynamic reproducible research documents/presentations. The development of team working skills, integral to this interdisciplinary field, will be achieved through group project work.
Following the semester two exam period, students will work full-time on their individual or group research project. At the end of the term, each student will submit a health data science research dissertation and give an oral presentation.
Curriculum Information
Curriculum information relates to the current academic year (in most cases).Course and module offerings and details may be subject to change.
Glossary of Terms
- Credits
- You must earn a defined number of credits (aka ECTS) to complete each year of your course. You do this by taking all of its required modules as well as the correct number of optional modules to obtain that year's total number of credits.
- Module
- An examinable portion of a subject or course, for which you attend lectures and/or tutorials and carry out assignments. E.g. Algebra and Calculus could be modules within the subject Mathematics. Each module has a unique module code eg. MA140.
- Subject
- Some courses allow you to choose subjects, where related modules are grouped together. Subjects have their own required number of credits, so you must take all that subject's required modules and may also need to obtain the remainder of the subject's total credits by choosing from its available optional modules.
- Optional
- A module you may choose to study.
- Required
- A module that you must study if you choose this course (or subject).
- Required Core Subject
- A subject you must study because it's integral to that course.
- Semester
- Most courses have 2 semesters (aka terms) per year, so a three-year course will have six semesters in total. For clarity, this page will refer to the first semester of year 2 as 'Semester 3'.
Year 1 (90 Credits)
OptionalST311: Applied Statistics I - 5 Credits - Semester 1OptionalST313: Applied Regression Models - 5 Credits - Semester 1
OptionalST413: Statistical Modelling - 5 Credits - Semester 1
OptionalST417: Introduction to Bayesian Modelling - 5 Credits - Semester 1
OptionalMA215: Mathematical Molecular Biology I - 5 Credits - Semester 1
OptionalMA284: Discrete Mathematics - 5 Credits - Semester 1
OptionalMA313: Linear Algebra I - 5 Credits - Semester 1
OptionalMA385: Numerical Analysis I - 5 Credits - Semester 1
OptionalCS4102: Geometric Foundations of Data Analysis I - 5 Credits - Semester 1
OptionalMD1602: Introduction to the Ethical and Regulatory Frameworks of Clinical Research - 10 Credits - Semester 1
OptionalEC5120: Economics of Health and Health Care - 10 Credits - Semester 1
OptionalEC584: Economic Evaluation in Health Care - 10 Credits - Semester 1
OptionalCT230: Database Systems I - 5 Credits - Semester 1
OptionalCT4101: Machine Learning - 5 Credits - Semester 1
OptionalCT5165: Principles of Machine Learning - 5 Credits - Semester 1
RequiredHDS5106: Health Data Science Research Project - 30 Credits - Semester 1
RequiredHDS5105: Statistical Computing for Biomedical Data - 5 Credits - Semester 1
RequiredHDS5102: Clinical Research Design - 5 Credits - Semester 1
RequiredHDS5104: Statistics for Health Data Science - 5 Credits - Semester 1
OptionalST312: Applied Statistics II - 5 Credits - Semester 2
OptionalMA216: Mathematical Molecular Biology II - 5 Credits - Semester 2
OptionalMA324: Introduction to Bioinformatics (Honours) - 5 Credits - Semester 2
OptionalMA461: Probabilistic Models for Molecular Biology - 5 Credits - Semester 2
OptionalMI439: The Meaning of Life: Bioinformatics - 5 Credits - Semester 2
OptionalMA203: Linear Algebra - 5 Credits - Semester 2
OptionalMA283: Linear Algebra - 5 Credits - Semester 2
OptionalMA378: Numerical Analysis II - 5 Credits - Semester 2
OptionalCS4103: Geometric Foundations of Data Analysis II - 5 Credits - Semester 2
OptionalCS4423: Networks - 5 Credits - Semester 2
OptionalMD515: Systematic Review Methods - 10 Credits - Semester 2
OptionalEC5130: Health Economic Analysis of Medical Devices - 10 Credits - Semester 2
OptionalCT5100: Data Visualisation - 5 Credits - Semester 2
OptionalST4140: Modern Statistical Methods - 5 Credits - Semester 2
RequiredHDS5103: Statistical Modelling for Health Data Science - 5 Credits - Semester 2
RequiredHDS5101: Predictive Modelling and Statistical Learning - 5 Credits - Semester 2
RequiredST4120: Causal Inference - 5 Credits - Semester 2
Why Choose This Course?
Career Opportunities
Data science skills are in unprecedented demand from many industries, particularly in Healthcare. From precision medicine, to next generation genomics, to individualised monitoring devices the growth in data collection and data-led decision making is revolutionising service delivery. Graduates of the MSc in Health Data Science will develop the key statistical and computing skills needed to design studies, analyse, interpret and translate research findings to evaluate health care interventions, services, programmes, and policies.
The graduates of this programme will be equipped with a skill set that is in high demand in the Healthcare, Pharma, MedTech, Biomedical, Sport Science and Health Insurance sectors as well as in academia.
Who’s Suited to This Course
Learning Outcomes
Transferable Skills Employers Value
Work Placement
Study Abroad
Related Student Organisations
Course Fees
Fees: EU
Fees: Tuition
Fees: Student levy
Fees: Non EU
For 25/26 entrants, where the course duration is greater than 1 year, there is an inflationary increase approved of 3.4% per annum for continuing years fees.
Postgraduate students in receipt of a SUSI grant – please note an F4 grant is where SUSI will pay €4,000 towards your tuition (2025/26). You will be liable for the remainder of the total fee. A P1 grant is where SUSI will pay tuition up to a maximum of €6,270. SUSI will not cover the student levy of €140.
Note to non-EU students: learn about the 24-month Stayback Visa here.
Find out More
School of Mathematical and Statistical Sciences
E: MSC.HDS@universityofgalway.ie
T: +353 91 492 332
www.universityofgalway.ie/courses/taught-postgraduate-courses/health-data-science.html